How SMEs Cut Document Processing Time by 80% with Automated Data Extraction
- Virtual Flow
- 22 hours ago
- 11 min read
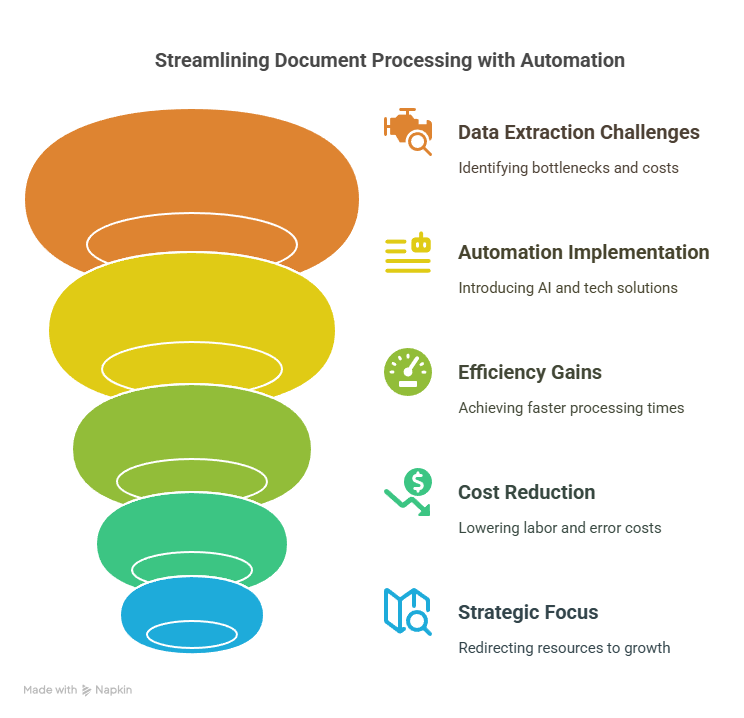
Document data extraction challenges cost SMEs countless hours and resources each year. Manually processing invoices, contracts, and compliance documents requires significant staff time, often leading to backlogs and operational bottlenecks that hinder business growth.
However, innovative document automation solutions are transforming this landscape. By implementing data extraction technologies and document AI systems, businesses are now converting unstructured information into structured data that seamlessly integrates with existing workflows. This digital transformation isn’t limited to large enterprises anymore—SMEs are particularly benefiting from these accessible tools, consequently reducing their document processing time by an impressive 80%.
This article explores three real-world case studies of SMEs that revolutionized their operations through automated data extraction. Additionally, we’ll examine how programmatic labeling techniques enable rapid iteration and deployment of these solutions, even for organizations with limited technical resources. Whether you’re struggling with KYC verification, complex financial documents, or risk assessment paperwork, these proven approaches demonstrate practical pathways to greater efficiency.
Manual Document Processing Challenges for SMEs
Small and medium enterprises struggle with inefficient document processing systems that drain resources and hinder growth. Beyond just being time-consuming, these manual processes create substantial operational challenges that affect the entire business ecosystem.
High Labor Costs in Manual Data Entry
Manual data entry remains a significant burden for SMEs, with research showing that up to 46% of supply chain professionals still rely on spreadsheets for operations [1]. This traditional approach demands excessive employee time, turning simple tasks into expensive processes.
The direct costs are substantial and straightforward to calculate. Consider a data entry clerk earning $15 per hour who takes 15 minutes to process a single document. This translates to $3.75 per document in labor costs alone [1]. Nevertheless, the true expense escalates when accounting for errors.
Data entry errors occur at alarming rates, ranging between 18% and 40% in typical scenarios [1][2]. These mistakes require correction, further increasing the cost per document to approximately $4.50 [1]. For a company processing just 100 documents daily, direct labor costs amount to $450 per day—not including the cascading effects of these errors [1].
Furthermore, manual data entry consumes over 40% of employee work hours that could otherwise be dedicated to strategic activities [2]. This represents a massive opportunity cost for businesses that could redirect these resources toward growth initiatives.
Inconsistent Document Formats and Layouts
As companies collect information from diverse sources, they face the challenge of handling inconsistent document formats. This inconsistency creates several operational difficulties that complicate data management processes.
First, integration becomes exceedingly complex when merging data from sources using different formats. This not only slows processing but also increases error risk during consolidation [3]. Moreover, teams must normalize or reformat data before analysis, creating additional processing steps that delay reporting and impact decision-making [3].
The quality of data often suffers when information from different sources is forced into uniform formats. This standardization attempt can lead to misinterpretation or loss of data granularity, potentially resulting in flawed business decisions [3]. Likewise, inconsistent formats create barriers to automation since automated systems rely on standardized inputs to function correctly [3].
Most SMEs lack standardized approaches to document management, resulting in files stored in different formats across various locations with inconsistent naming conventions [4]. This disorganization leads to information silos, duplication of work, and missed deadlines [4].
Delayed Turnaround Times in Business Workflows
Processing delays represent one of the most visible impacts of manual document handling. According to industry analysis, companies with inefficient processes experience 30-50% longer cycle times alongside higher labor costs [5].
The state-level filing process demonstrates these delays clearly. Normal processing times for business filings vary dramatically by state—from 14 days in most states to as much as 180 business days in Alabama [6]. Even with expedited services, processing can still take several days to weeks [6].
These delays extend beyond just document processing. The majority of manufacturers report work delays related to inventory data (62%), manufacturing throughput times (62%), and equipment effectiveness (62%) [7]. Such bottlenecks directly impact a company’s ability to compete effectively in today’s market [7].
In legal and financial sectors, processing delays frequently lead to client dissatisfaction. One case study reported a law firm losing a top retainer client due to regular delays in communication despite having talented attorneys and support staff [5]. The absence of standardized processes created bottlenecks and miscommunications that damaged the relationship irreparably.
The problems most frequently reported by SMEs include deficiencies in document organization, information loss, and delayed document requests [3]. These issues compound over time, affecting client-facing work and ultimately hindering business growth [5].
Case Study 1: Reducing KYC Processing Time by 80%
Financial institutions face mounting pressure to streamline Know Your Customer (KYC) procedures while maintaining strict regulatory compliance. One major U.S. bank successfully transformed this challenging process through advanced document data extraction technology.
Attribute Extraction from 10-Ks Using Snorkel Flow
Initially, the bank struggled with extracting critical information from Form 10-K filings—lengthy annual reports often exceeding 300 pages. These documents contain essential company data required for thorough KYC verification. Prior to automation implementation, employees manually scoured these complex documents to locate and extract key information [8].
The solution came through Snorkel Flow, a platform specializing in programmatic labeling and automated data extraction. The system was configured to automatically identify and extract approximately 15-20 critical attributes from each 10-K document, including:
Company name and headquarters location
Nature of business operations
Board of directors composition
Senior management identification
Total asset valuation
Financial performance indicators [1]
This technological approach eliminated the need for manual searching through hundreds of pages, essentially transforming unstructured document data into structured, analyzable information. The extraction model achieved impressive accuracy rates exceeding 89% [8], significantly surpassing previous manual methods in both speed and reliability.
Replacing 500 Analysts with Programmatic Labeling
Before implementing automated extraction, the bank relied on a team of 300-500 KYC analysts who manually reviewed each document [8]. This labor-intensive approach created substantial operational bottlenecks despite the significant workforce allocated to the task.
Programmatic labeling fundamentally changed this paradigm. Unlike traditional manual labeling, which requires item-by-item tagging, this approach employs rules-based functions that can label large datasets rapidly. The system uses heuristic patterns and external knowledge bases to identify relevant information across multiple documents concurrently [8].
The most remarkable aspect of this transformation was the ability to process documents continuously without interruption. While human analysts required breaks and worked standard hours, the automated system operated 24/7, subsequently reducing the overall processing timeline by approximately 80% [9]. This dramatic improvement allowed the bank to increase client onboarding capacity substantially while maintaining compliance with increasingly complex regulations.
10,000 Person-Hours Saved Annually
The quantifiable benefits of this automation project proved substantial. With analysts previously spending between 30-90 minutes reviewing each document and processing over 10,000 reports annually, the bank reclaimed approximately 10,000 person-hours each year [1].
This time savings translated to an estimated $500,000 in direct labor cost reduction [8]. Notably, these figures represent only the immediate financial impact—they don’t account for the additional benefits of improved accuracy, faster customer onboarding, and reduced regulatory risk.
The bank also gained valuable agility in responding to changing regulations. Programmatic labeling allowed the KYC teams to collaborate effectively with data science departments, rapidly updating extraction parameters whenever regulatory requirements changed [10]. This adaptability proved particularly valuable during periods of regulatory evolution in the financial sector.
The remarkable reduction in processing time—from weeks to days—ultimately transformed the customer experience while strengthening compliance effectiveness. By automating this critical function, the bank not only addressed existing challenges but also created capacity for addressing more complex KYC issues requiring human judgment and expertise [10].
Case Study 2: Automating Interest Rate Swap Extraction
Interest rate swaps constitute the largest segment of the global over-the-counter derivatives market, representing an astonishing $372.00 trillion in notional value—twenty-five times larger than the U.S. public equities market [1]. For financial institutions, extracting this critical information from complex documentation poses substantial challenges that conventional document processing methods struggle to address.
Labeling 70,000 Data Points with Labeling Functions
A top-3 U.S. bank found that manually reviewing 10-K filings for interest rate swap data required 40-45 analysts working 12-15 hours weekly [1]. Recognizing this inefficiency, the bank implemented Snorkel Flow’s programmatic labeling approach to automate document data extraction.
Instead of traditional manual labeling, where analysts tag data points individually, programmatic labeling uses defined functions that automatically identify and categorize information. This approach enabled the labeling of approximately 70,000 data points [1]—a scale that would have been practically impossible through conventional methods.
The programmatic labeling process functions through:
Writing heuristic-based labeling functions via a GUI or Python SDK
Automatically generating labels from small labeled samples
Executing these functions across massive unlabeled datasets
Aggregating results through advanced algorithms that resolve conflicting labels [11]
This methodology ultimately generates weak labels at remarkable speeds—up to 70,000 labels per minute [1]—dramatically outpacing human capabilities.
Handling Table Variations and Textual Mentions
Perhaps the greatest challenge in automating interest rate swap extraction stems from inconsistent data presentation throughout documents. The information might appear:
In tables listing notional amounts, sometimes broken down by years or quarters
Within text paragraphs stating that the company entered specific swap agreements
As multiple separate amounts distributed throughout the document
In tables with varying structures, column counts, and formatting [1]
Accordingly, the document automation system needed to recognize multiple presentation formats. The programmatic approach proved especially valuable because traditional rule-based extraction struggles with such variations [12].
Cross-page tables presented additional complexity, as information often spanned multiple pages with repeating headers or continuation cells [12]. The implementation used specialized functions to identify and correctly merge these fragmented data presentations, ensuring complete information extraction regardless of document layout.
Reducing Analyst Time from 263 Hours to Minutes
The impact on processing efficiency proved remarkable. In one representative project, what previously required a dozen analysts investing 263 total hours [1] was reduced to a task that could be completed in minutes.
Following implementation, analysts could focus on reviewing high-confidence extractions instead of performing tedious manual searches. First thing to remember is that this shift represented not merely incremental improvement but a fundamental transformation in workflow efficiency.
Beyond time savings, the approach delivered additional benefits:
Improved data accuracy through consistent extraction methodologies
Enhanced compliance capabilities through comprehensive documentation coverage
Greater ability to identify trends across company filings and time periods
In essence, the automated document data extraction system enabled the bank to transform unstructured financial information into structured data that could be easily analyzed and incorporated into existing workflows—creating both operational efficiency and strategic advantage.
Case Study 3: Risk Factor Extraction for Investment Monitoring
Risk management professionals confront massive volumes of unstructured text within financial documents that contain critical information about potential threats to investments. A leading banking customer implemented an automated risk factor extraction system that transformed how they monitor investment risks.
Extracting Paragraphs from Unstructured 10-Ks
The SEC requires public companies to disclose risk factors in Form 10-K filings, typically presenting 15-50 risk factors annually [3]. These disclosures outline potential threats to company performance, yet exist as unstructured paragraphs buried within massive documents. Traditionally, analysts manually read through entire filings to identify relevant risk information—an approach that proved unsustainable given the volume of 10-Ks filed each year.
The solution employed programmatic labeling to automatically extract risk factor paragraphs from unstructured documents and present them in structured formats. This approach analyzed sentence skeletons by recognizing noun phrases based on part-of-speech tagging [5]. The system then mapped each extracted paragraph to specific risk categories, including financial, strategic, operational, and hazardous risks [5].
Trend Analysis Across Industries and Time
Once extracted, risk factors became available for sophisticated analytical applications. Risk profiles could be generated from the 10-K data, with each profile containing measures for different types of risks [5]. On average, companies disclosed 254 risk sentences in 10-Ks (strategic: 160, financial: 87, operational: 6, hazardous: 1) [5].
This structured format enabled analysts to identify trends across companies, industries, and time periods. By tracking changes in risk factor disclosures year-over-year, the system revealed emerging threats that might otherwise remain hidden. Companies typically add only 1-5 new risk factors annually while reordering existing ones [3], making systematic tracking essential for identifying subtle shifts in risk landscapes.
Triggering Risk Alerts for Analysts
Perhaps most valuable was the system’s ability to automatically flag new or intensified risks. When a particular risk factor appeared in a company’s 10-K that was absent in previous years, the system generated alerts for investment analysts [1]. Similarly, if risk factors changed position (appearing earlier in the disclosure), this could signal increased importance [3].
Through this approach, the bank saved hundreds of analyst hours while simultaneously improving risk monitoring capabilities [1]. The resulting application provided resilient, adaptable risk intelligence that continuously improved as more documents were processed.
How Programmatic Labeling Enables Rapid Iteration
Programmatic labeling stands as the cornerstone technology behind the remarkable efficiency gains demonstrated in the previous case studies. This approach fundamentally changes document data extraction by letting users create, refine, and deploy automated extraction systems rapidly with minimal technical overhead.
Writing Labeling Functions with GUI or Python SDK
Labeling functions serve as the basis for automated data extraction, essentially acting as rules that match patterns within documents. Users can create these functions through:
The key advantage lies in speed—instead of manually tagging individual data points, labeling functions can generate up to 70,000 labels per minute [1]. This exponential efficiency gain allows SMEs to create comprehensive extraction systems with minimal resource investment.
De-noising Conflicting Labels Automatically
As multiple labeling functions operate simultaneously, they inevitably produce conflicting results. Henceforth, advanced algorithms automatically resolve these conflicts through:
Evaluation of each function’s reliability across datasets
Detection of correlation patterns between functions
Aggregation of weak labels into strong labels via statistical methods [13]
This “weak supervision” approach effectively combines noisy signals from various labeling functions into highly accurate training labels without extensive manual intervention [14]. The system learns which functions to trust in which contexts, thus delivering increasingly accurate results through continued use.
Training Models and Performing Error Analysis
After generating labels, the system trains machine learning models that generalize beyond explicit rules. Thereafter, sophisticated error analysis tools guide users to improve both models and labeling functions by:
This iterative analysis creates a continuous improvement cycle where each round of refinement improves extraction accuracy. Besides accuracy improvements, this approach yields significant adaptability—when document formats or extraction requirements change, users can quickly modify labeling functions and regenerate the entire system within minutes rather than rebuilding from scratch [11].
Conclusion
Automated data extraction technology has fundamentally transformed document processing for SMEs, delivering remarkable efficiency gains while reducing operational costs. Throughout these case studies, we’ve seen dramatic reductions in processing times—consistently reaching 80% improvement—while simultaneously enhancing accuracy and compliance capabilities. Consequently, businesses previously drowning in paperwork now redirect valuable human resources toward strategic initiatives rather than tedious manual tasks.
The financial impacts prove equally impressive. Companies implementing these solutions reclaim thousands of person-hours annually, translating to hundreds of thousands in direct cost savings. Undoubtedly, these technologies democratize access to advanced document processing capabilities previously available only to large enterprises with substantial IT budgets.
Most compelling evidence comes from the versatility demonstrated across different document types. From complex KYC procedures to intricate financial derivatives documentation and risk factor analysis, programmatic labeling adapts to virtually any document challenge. This adaptability makes these solutions particularly valuable as business requirements evolve and document formats change.
Overall, automated data extraction represents a critical competitive advantage for forward-thinking SMEs. The ability to rapidly convert unstructured information into actionable data accelerates decision-making while reducing error rates. Additionally, these systems become more effective over time through continuous iteration and refinement, creating sustainable long-term value.
The path toward implementation has also become significantly more accessible. As demonstrated through programmatic labeling approaches, companies can now develop sophisticated extraction systems without extensive technical expertise or massive resource commitments. This accessibility essentially removes the last major barrier to adoption for small and medium businesses seeking operational excellence through document automation.
References
[1] - https://snorkel.ai/blog/10-ks-information-extraction-case-studies/[2] - https://www.uxopian.com/blog/solving-common-document-processing-challenges[3] - https://www.researchgate.net/publication/228236811_Exploring_the_Information_Contents_of_Risk_Factors_in_SEC_Form_10-K_A_Multi-Label_Text_Classification_Application[4] - https://info.docxellent.com/blog/document-management-tips-for-small-medium-enterprises[5] - https://www.cpajournal.com/2020/07/15/textual-analysis-for-risk-profiles-from-10-k-filings/[6] - https://labelstud.io/blog/5-tips-and-tricks-for-label-studio-s-api-and-sdk/[7] - https://www.sme.org/aboutsme/newsroom/press-releases/2023/manufacturers-challenged-with-manual-operations-and-work-delays--according-to-sme-and-laserfiche-study/[8] - https://emerj.com/ai-for-kyc-regulations-use-cases/[9] - https://www.rpatech.ai/kyc-automation-top-10-benefits/[10] - https://snorkel.ai/blog/data-extraction-sec-filings-10-ks/[11] - https://snorkel.ai/data-centric-ai/programmatic-labeling/[12] - https://techcommunity.microsoft.com/blog/azure-ai-services-blog/a-heuristic-method-of-merging-cross-page-tables-based-on-document-intelligence-l/4118126[13] - https://snorkel.ai/data-labeling/[14] - https://pmc.ncbi.nlm.nih.gov/articles/PMC7484266/[15] - https://medium.com/@ktoprakucar/a-comprehensive-guide-to-error-analysis-in-machine-learning-288e353f7c8d[16] - https://docs.snorkel.ai/docs/25.3/user-guide/analysis/creating-good-labeling-functions/
Comments